Generative AI in Business
- Telescope Team
- May 17
- 5 min read
The AI revolution is in full swing, and companies across the globe are exploring how generative AI can drive transformation. This technology represents a major leap in the way humans engage with machines, and it has the potential to disrupt nearly every industry.
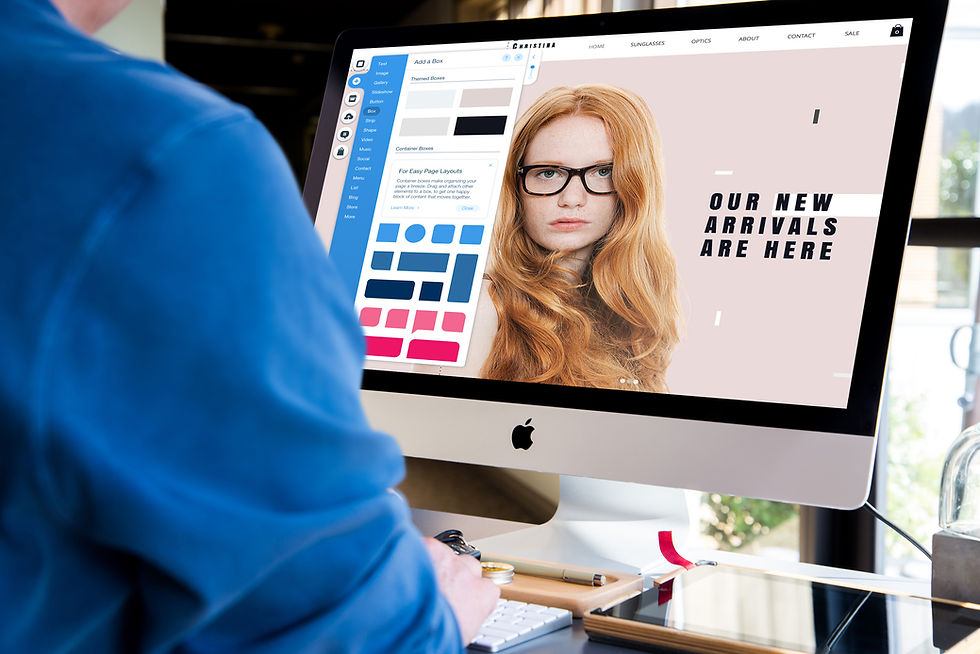
Understanding Generative AI
Technological history is punctuated by groundbreaking moments that alter entire industries, change how we engage with devices, and reshape society. Think of the launch of the Netscape browser in 1994 or the introduction of the iPhone in 2007—generative AI marks a similarly significant milestone. While tools like ChatGPT have wowed consumers by producing polished text and visuals in seconds, the real game-changer lies in what generative AI can do for businesses. Leaders across sectors are now actively exploring how to implement this technology throughout their operations. Companies like Koch Industries and institutions like the U.S. Air Force, which have long invested in enterprise AI, now see generative AI as a way to supercharge digital transformation. The potential gains are massive, and the cost of inaction could be equally large.
What Sets Generative AI Apart?
Generative AI, as the name implies, enables computers to create new content using advanced AI and machine learning algorithms trained on massive datasets. Its conversational interface represents a major evolution in human-computer interaction. This technology allows software to understand and replicate the patterns in images, text, and audio—generating detailed and comprehensive outputs in seconds, though occasional errors still occur.
Technologies like transformer models, generative adversarial networks (GANs), and variational autoencoders make this possible. Today, beyond ChatGPT, tools like Stability.ai, Google Bard, and Microsoft’s AI-powered Bing (“Ask real questions. Get complete answers.”) are reshaping how content is created. These tools rely heavily on public data, which is useful for general purposes—but enterprises need more.
What truly unlocks generative AI’s value for businesses is access to internal, proprietary data. A bank, for instance, doesn’t benefit much from a model trained only on public datasets. It needs a domain-specific AI system that draws insights from its private information—such as customer deposit trends or loan data. The same logic applies to sectors like manufacturing and healthcare. While public data still plays a role through Large Language Models (LLMs), it’s the enterprise-specific data that turns generative AI into a powerful business tool.
The benefits don’t end there. Generative AI democratizes access to advanced analytics, allowing more employees—not just data scientists—to tap into the predictive capabilities of enterprise AI. Public-facing generative AI creates responses based on past, internet-wide information. In contrast, enterprise-grade generative AI delivers actionable forecasts tailored to a company’s own operations—like predicting equipment failures or identifying customers likely to churn.
Key AI Terms and Definitions
Before exploring the value of generative AI in enterprise settings, it’s helpful to understand several foundational terms:
Natural Language Processing (NLP): A field of AI focused on enabling machines to comprehend, analyze, and generate human language.
Transformer: A groundbreaking neural network design introduced by Google in 2017. It made it possible to train massive models on vast datasets, laying the foundation for today’s large language models (LLMs) by identifying patterns and relationships in sequences of text.
Large Language Model (LLM): These are advanced deep learning systems built to generate text. Most modern LLMs are based on the transformer architecture. What sets them apart is their enormous size—typically billions of parameters—and the extensive textual data used for training.
Examples of closed-source/commercial LLMs: OpenAI's GPT-3/3.5/4, Google Cloud's PaLM, AWS Bedrock, Anthropic's Claude.
Examples of open-source models: Google FLAN-T5 & FLAN-UL2, MPT-7B, Falcon.
Pre-trained/Foundational Model: These models are developed using self-supervised learning (without human-labeled data) on vast datasets. They're intended as a starting point and require further tuning for specific applications.
Fine-tuning: This is the process of adapting a foundational model to a targeted task (e.g., summarization or Q&A) using labeled data. It’s far more efficient than training from scratch and can result in high-performing models even with less computational power.
Hallucination: When an LLM produces inaccurate or fabricated information. This typically happens because the model generates responses based on its training data, which might not align with the user’s query or real-world facts.
Multi-modal AI: AI systems that can interpret and respond to various input types—text, audio, images, and video.
Retrieval Model: A tool (often a type of transformer) that locates relevant information from a database or knowledge base. When combined with LLMs, retrieval models help reduce hallucinations by grounding responses in reliable data.
Vector Store: A specialized database for storing and retrieving vector embeddings of documents. These stores are optimized for similarity search, playing a key role in generative AI architectures.
Enterprise AI: AI systems designed to offer predictive insights that help improve core business processes and decision-making.
Generative AI in Enterprise Search: Applying generative AI to enhance search functionality across enterprise data and analytics, making complex insights accessible to all users, not just technical teams.
How Generative AI Is Revolutionizing Enterprises
Generative AI can dramatically increase productivity by creating high-quality, relevant content—be it text, visuals, or video—far more quickly than traditional methods. This capability is reshaping a broad range of industries, from manufacturing and healthcare to finance, government, and retail.
For example:
Marketers can produce a wide variety of personalized content without increasing headcount.
Financial professionals can quickly generate detailed, customized reports for stakeholders.
These improvements drive significant cost savings, enhance customer experiences, and accelerate business outcomes.
A particularly transformative use case is enterprise search. Imagine a search engine tailored for your business that gives instant access to the most relevant data, reports, and predictive insights. Instead of relying solely on data analysts, any employee can use a simple prompt to unlock deep organizational knowledge. This democratization of data leads to smarter, faster decisions across all departments.
Example: Supporting Machinery Operators with Generative AI
Consider a machine operator overseeing production equipment. Their responsibilities include monitoring performance, handling alerts, and ensuring the line meets output and quality goals. These tasks are demanding, and operators rarely have time to sift through manuals or analyze complex data.
Many manufacturers also face a knowledge gap as experienced staff retire. Generative AI offers a solution by capturing and synthesizing institutional knowledge. An LLM trained on internal documents—like maintenance logs, performance reports, and OEM manuals—can assist operators in real time.
Let’s say the conveyor belt on Line A stops working. An operator types into the AI interface: "How do I fix the conveyor belt on Line A?" The system instantly returns step-by-step troubleshooting instructions from the machine’s SOP, along with relevant notes from past work orders.
This approach empowers newer operators with the insights of seasoned experts—without requiring years of experience. The AI distills vast, scattered data into clear, actionable information through a natural language interface. As a result, operators can resolve issues faster, improve reliability, and ultimately drive better business performance.
Comments